
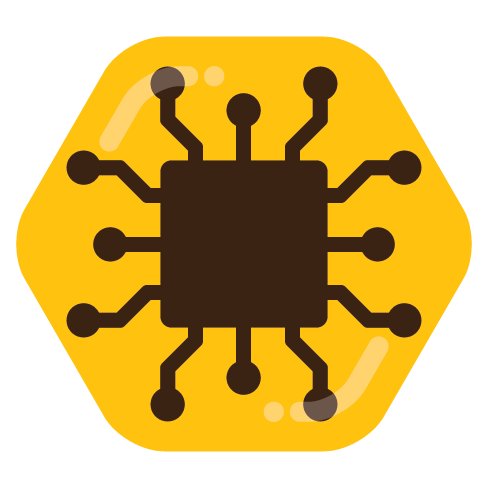
This isn’t my field, and some undergraduate philosophy classes I took more than 20 years ago might not be leaving me well equipped to understand this paper. So I’ll admit I’m probably out of my element, and want to understand.
That being said, I’m not reading this paper with your interpretation.
This is exactly what they’ve proven. They found that if you can solve AI-by-Learning in polynomial time, you can also solve random-vs-chance (or whatever it was called) in a tractable time, which is a known NP-Hard problem. Ergo, the current learning techniques which are tractable will never result in AGI, and any technique that could must necessarily be considerably slower (otherwise you can use the exact same proof presented in the paper again).
But they’ve defined the AI-by-Learning problem in a specific way (here’s the informal definition):
Given: A way of sampling from a distribution D.
Task: Find an algorithm A (i.e., ‘an AI’) that, when run for different possible situations as input, outputs behaviours that are human-like (i.e., approximately like D for some meaning of ‘approximate’).
I read this definition of the problem to be defined by needing to sample from D, that is, to “learn.”
The explicit point is to show that it doesn’t matter if you use LLMs or RNNs or whatever; it will never be able to turn into a true AGI
But the caveat I’m reading, implicit in the paper’s definition of the AI-by-Learning problem, is that it’s about an entire class of methods, of learning from a perfect sample of intelligent outputs to itself be able to mimic intelligent outputs.
General Intelligence has a set definition that the paper’s authors stick with. It’s not as simple as “it’s a human-like intelligence” or something that merely approximates it.
The paper defines it:
Specifically, in our formalisation of AI-by-Learning, we will make the simplifying assumption that there is a finite set of possible behaviours and that for each situation s there is a fixed number of behaviours Bs that humans may display in situation s.
It’s just defining an approximation of human behavior, and saying that achieving that formalized approximation is intractable, using inferences from training data. So I’m still seeing the definition of human-like behavior, which would by definition be satisfied by human behavior. So that’s the circular reasoning here, and whether human behavior fits another definition of AGI doesn’t actually affect the proof here. They’re proving that learning to be human-like is intractable, not that achieving AGI is itself intractable.
I think it’s an important distinction, if I’m reading it correctly. But if I’m not, I’m also happy to be proven wrong.
Well, also, these are documents written in the past, before 1948, when the Supreme Court invalidated the effect of racial covenants.
But the language remains, with no legal effect. But it’s still there and should be eliminated. There’s no cat and mouse game, just the need for cleanup of something left from the past.